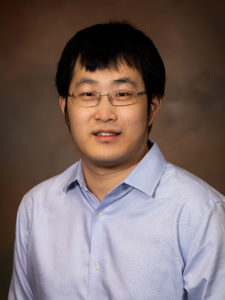
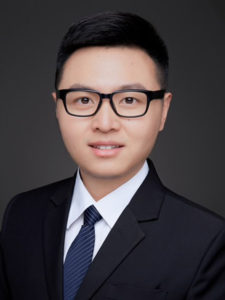
Electrical and computer engineering assistant professors Weilu Gao and Cunxi Yu recently published a paper in Nature Computational Science, a multidisciplinary journal focused on the development and use of computational techniques and mathematical models as well as their ability to address complex problems across a range of scientific disciplines. Their paper, “Physics-informed recurrent neural network for time dynamics in optical resonances,” introduces a high-performing machine-learning algorithm to accelerate data acquisition times of optical resonance without losing analysis accuracy. Their work has also been released publicly as an open-source project.
Optical resonance structures and features are central to many areas of optical science research and exhibit exciting potential for a broad range of innovations in diverse disciplines like health sensing and imaging, security, computing, communication and quantum technology. Current methods for capturing and understanding the time-domain signals of optical resonance features, however, are time-consuming or have low analysis accuracy.
“As a postdoc, I remember conducting experiments with long data acquisition times that took days to weeks and wondering if there was a way to accelerate the process. As an assistant professor I was reminded of this problem and began collaborating with assistant professor Yu to implement machine learning techniques to speed up the task,” Gao said.
Yu and Gao’s algorithm can accurately predict the time-domain response of optical resonances and infer corresponding resonance frequencies by acquiring a fraction of the sequence as input. Instead of directly training the model using data obtained from time-consuming electromagnetic calculations or experiments, the two employ a two-step framework that first uses synthetic data generated by a physical model and then a small set of application-specific data.
Through simulations and experiments, Yu and Gao demonstrate that for certain applications, their model is up to eight times shorter than current methods and can be applied to a wide range of resonances. Their work will help to accelerate the exploration of physical phenomena and device design under resonance-enhanced light-matter interaction.
Read Yu and Gao’s full paper here.